Accueil
Data Mining in Finance: Advances in Relational and Hybrid Methods
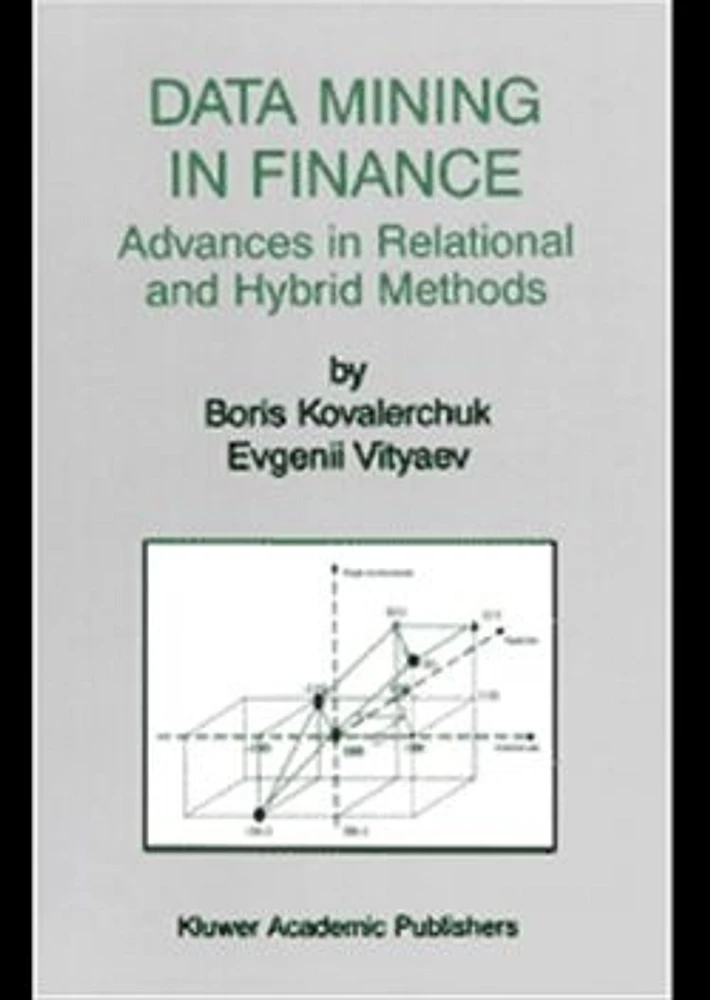
LIBRAIRIE CARCAJOU
Data Mining in Finance: Advances in Relational and Hybrid Methods
De Librairie Carcajou
This overview of major algorithmic approaches to predictive data mining, includes statistical, neural networks, ruled-based, decision-tree, and fuzzy-logic methods. It examines the suitability of these approaches to financial data mining. The book focuses specifically on relational data mining (RDM), which is a learning method able to learn more expressive rules than other symbolic approaches. RDM is thus better suited for financial mining, because it is able to make greater use of underlying domain knowledge. Relational data mining also has a better ability to explain the discovered rules - an ability critical for avoiding spurious patterns which inevitably arise when the number of variables examined is very large. The earlier algorithms for relational data mining, also known as inductive logic programming (ILP), suffer from a relative computational inefficiency and have rather limited tools for processing numerical data. The approach expounded here combines relational data mining with the analysis of statistical significance of discovered rules.